국제 학술 세미나
언론정보학 해외저명학자 초청 특강: 10/8(화) 이부형: An exploratory study of time series analysis in communication research
일자
2024.10.08
작성자
admicr
작성일
2024-11-20
조회
684
일시: 10월 8일 (화) 12:30 - 14:00
장소: 서울대학교 64동 (IBK커뮤니케이션센터) 501호
강연자: 이부형 교수님 (Loyola University Maryland)
제목: An exploratory study of time series analysis in communication research
내용:
A time series is a sequence of data collected or recorded chronologically, usually at uniform intervals. We encounter this type of data in various fields, including economics/finance (e.g., daily closing stock prices, monthly price indices, quarterly unemployment rates), meteorology (e.g., hourly temperature/precipitation/wind speed), and engineering (e.g., sound waves, electric signals). By examining those sequential measurements over time, researchers can identify trends, patterns, and relationships that might not be apparent from a single snapshot or sample. In addition, time series analysis can be a powerful tool in communication research, for example, to illustrate how legacy/social media’s attention to specific issues fluctuates over time and to demonstrate how changes in media content influence public opinion or political/commercial campaigns over time. Particularly, in case the time series is characterized by a linear relationship between current and past values (so-called autocorrelation), ARIMA (Autoregressive Integrated Moving Average) models can be suitable for understanding and predicting the time-dependent media phenomenon and effects. In this talk, I will review fundamental concepts in time series analysis and discuss ARIMA applications in communication research based on two real examples selected from recent communication publications.
강연자 소개: Bu Hyoung Lee is a statistician and an associate professor of statistics at Loyola University Maryland in the United States. He earned an M.A. degree in communication from Seoul National University in 2006 and a Ph.D. degree in statistics from Temple University in 2016. His research interests include spatial/temporal data analysis and machine learning with complicated/incomplete data.
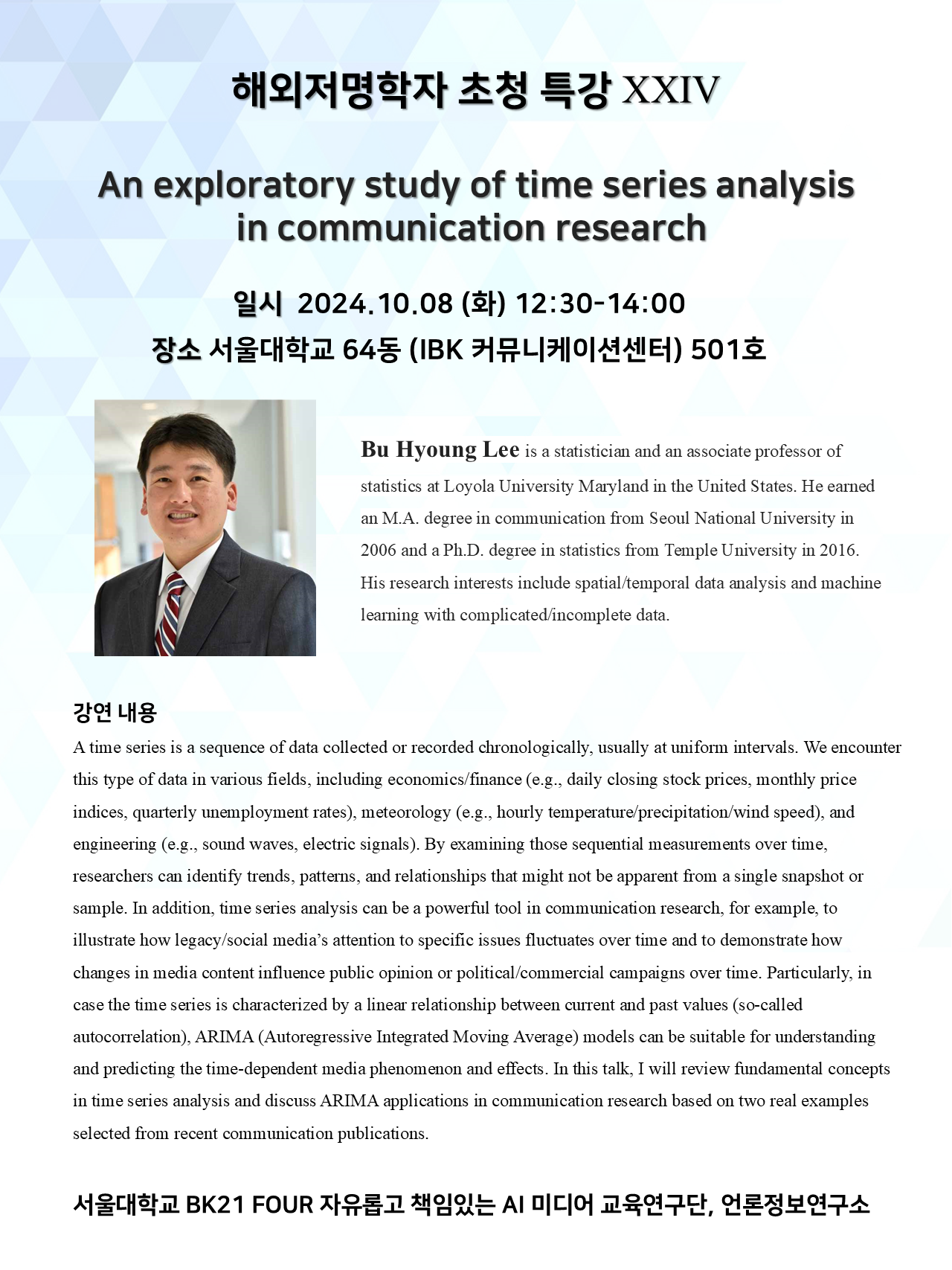